AI Software to Evaluate Brain Maturity Model
March 17, 2021
Researchers at Finland’s University of Helsinki and Helsinki University Hospital (HUH) have utilised artificial intelligence (AI) to create machine-learning software that can decode EEG data from a preterm child and determine the brain’s functional maturity without any human intervention.
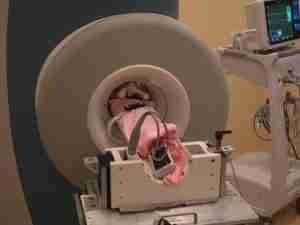
This methodology, which was just published in Scientific Reports, is the first of its kind to use electroencephalographic (EEG) data to evaluate brain development. It provides automatic and quantitative observation of a preterm infant’s brain growth and is now more effective than other techniques of evaluation.
Automation and Artificial Intelligence
Currently, we use growth charts to keep tabs on things like a birth development, size, and head size as they develop. Keeping tabs on a preemie’s neurological development is a realistic task made easier with the use of EEG monitoring coupled with computerised analysis.
Read Also: Brain Reading as The Mind Reading Technology
Professor Samplasa Vanathalo of the University of Helsinki, who oversaw the study, said that the findings “will provide knowledge that will assist plan the most effective treatment for the particular kid.”
In the words of the researchers, “this technology gives us the first chance to follow the most essential growth of a core child, the operational growth of the mind, both during and following ventilator.”
Machine Learning Programs
Premature birth affects around 10% of all births, and accounts for over 50% of all newborns requiring acute care. The fetus’s electrical brain activity changes practically weekly in the last stages of pregnancy, a period of fast AI software development. The correct growth of the brain depends on its ability to perform.
Artificial intelligence software may help overcome many of the health challenges connected with premature delivery. In the 1980s, scientists discovered that preterm newborns’ poor brain development in the initial months was a major cause of their health issues.
We need to understand how newborn brain functions mature so that we can give optimal care and create novel treatments, but there are currently no reliable and objective ways for assessing the quality of early artificial intelligence applications.
Using an EEG sensor attached to the scalp is the most desirable method for gauging mental development. In the last several years, tracking AI software in newborns critical care units has become more common since it is non-invasive, inexpensive, and has no inherent risks.
The analysis of EEG data is time-consuming and needs the physician doing the monitoring to have specific training. Vanhatalo asserts that this issue may be resolved worldwide and reliably by the use of automatic classification as element of an EEG equipment.
Helpful technical data and instructions for installing machinery in the PRETERM
In Professor Vanathelo’s lab, Australian engineer Nathan Stevenson worked as a Marie Curie Fellow supported by the European Union to develop novel software for analysing electrical brain activity. The study made use of EEG data collected of premature newborns that had been obtained under strict conditions by the lab on Professor Katrin Klebermas somewhere at Medical University of Vienna.
Machine learning is at the core of analysis software. Massive amounts of EEG data from premature newborns were loaded into a supercomputer, and without human interaction, the programme determined hundreds of computational characteristics from each measurement.
These features were used in conjunction with a linear kernel to arrive at an accurate prediction of the newborn’s EEG specific age. At the conclusion of the trial, the software was evaluated by comparing its prediction of the newborn’s EEG maturational age with the infant’s actual age, as determined by clinical assessment.
An infant’s actual age and the computer’s estimate would be within seven days of one another during over 80% of instances. Due to the high reliability and accuracy of the maturity assessment, the structural progression of the brain can be traced in all of the 39 newborns included in the research by repeating the measurements at regular intervals.
Methodologies in Artificial Intelligence Software
In order to guide tailored therapy and serve as a standard for emerging interventional research aimed at tracking early neurological development, invasive procedures, robotic cot-side instruments may be quite useful. To begin, we create an algorithm for a fully-automated assessment of the baby’s EEG specific age (EMA) using serial recordings.
The EMA was calculated using a mixture of 23 computational characteristics evaluated during both quiet and active EEG states (46 features in total). Training data for the mixture function (logit model) included 101 serial EEG recordings from newborns with a healthy developmental performance at twelve months old and a gestational age of fewer than gestation. From 24 to 38 weeks PMS, participants’ EEGs were recorded (PMA).