Machine Learning and Improving Clinical Trials
Today, a new study from UCL released in the neuroscience reveals that machine learning may enhance our capacity to evaluate whether a new treatment is working in the brains, possibly allowing researchers to uncover pharmacological properties that will be entirely overlooked by standard statistical analyses.
“At the moment, statistical models are really basic. They can’t reliably detect subtle but important biological differences between individuals, dismissing them as random variation. We reasoned that this could be a reason why many drug tests show positive results in very basic animals.
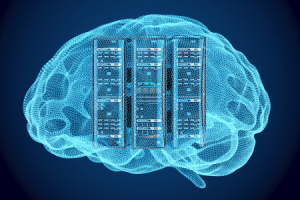
But they fall short when applied to the sophisticated human brain. If that’s the case, machine learning with the ability to simulate the brain activity in all its complexity might reveal therapy effects that were previously unknown.
Application for Coordinating Clinical Trials
In order to put the theory to the test, the study team examined huge amounts of data from post – stroke, identifying intricate morphological patterns of neurological destruction produced by strokes in each person, creating the biggest collection of anatomically recorded pictures of stroke to date. Sometimes went poorly. Patients’ gaze directions were assessed on CT scans of the head taken upon hospital admission, usually between one and three days after an MRI was performed, and were used as an indicator of the stroke’s impact.
They then pretended to conduct a massive meta-analysis of a group of fictitious medications in order to determine whether or not machine learning could reliably identify treatment effects of varying magnitudes that had been missing by conventional statistical methods. They showed a substantial impact using both classic (low-dimensional) statistical tests and high-dimensional machine learning approaches, for instance, when given a medication therapy that decreased brain abnormalities by close to 70%. evaluated by means of testing.
Python and Machine Learning
Stroke was conceptualised as a complicated “fingerprint,” defined by a number of characteristics, while machine learning approaches considered whether or not damage occurred across the brain.
“Stroke tests employ very modest, raw data, including the depth of the incision, and do not take into account whether the incision is centred on a vital location or is located towards the periphery. Instead, using hundreds of characteristics at strong health granularity, our programme learnt the whole extent of injury thru out the mind.
Lead author Tianbo Xu (UCL Institute of Neurology) said, “It allowed us to evaluate therapy effects with significantly higher sensitivity than previous approaches, highlighting the complicated link among anatomical structures and clinical results.”
education in the use of machine learning
The benefits of using machine learning to analyse treatments to prevent wounds were most obvious when comparing different methods. However, the high-dimensional model is likely to detect an impact higher compared to when the lesion is only 55 Had decreased to%, while the previous low-dimensional model would need the injury to compress up to 78.4% of its size for the impact to be observed in more frequent testing.
“Even if the treatment normally halves the severity of the stroke, or more, standard predictive methods will lack an impact because the intricacy of the mind’s overall function is evaluated clinically, and this is where the error occurs.
The AI vs. ML Debate
Increases the importance of individual success in determining the outcome. Although saving half of the damaged region of the brain may not have a noticeable effect on the patient’s behaviour, it is still worth doing so. Dr. Nachev once observed, “There is absolutely no such thing as an unneeded brain.
The real worth of cognitive computing lies in its ability to formalise very complicated judgments, rather than in its ability to automate mundane tasks. An individual doctor’s natural adaptability may be combined with the rigidity of data that propels evidence-based treatment via the use of machine learning. Even when taking into account several variables, a model’s language may remain dry and mathematical. With this new method, we can accurately record the intricate connection between anatomy and result, as Dr. Nachev put it.
Read Also: Experts Call for Rules to Protect Privacy Law
Research in Artificial Intelligence
Author Professor Geraint Rees hopes that “researchers and physicians start applying our methodologies the next moment we are required to organise a clinical study” (Dean, UCL Faculty of Life Sciences).
Even if the population as a whole reveals the incredibly complex functional architecture of the human mind research, the opposite inference, from efficient infrastructure mapping to personal behavior, is limited by the pronounced individual variances from the mean.
A Guide to Machine Learning
In localised head trauma, at which impact of an acquired systemic change in the head is decided by the implications of its behaviour, this conclusion is crucial for assessing the efficacy of therapeutic approaches. Has its path bent around an obstacle.
Current medical assessments do not take into account unique outcomes obtained from a full explanation of the anatomical structure of the tumours, instead relying on simplifications such the lesion volume and the location of the lesion after extensive dissection.