Fight Cancer With Open Source Machine Learning
This is a darn near perfect setup for theft. Open – sourced Reinforcement Learning, which employs artificial intelligence and raw genetic material to forecast the efficacy of cancer therapy, does the same for disease warriors and serves as a source of inspiration for them.
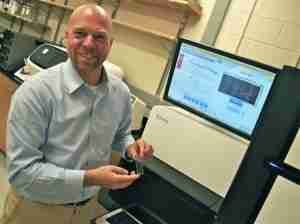
The program’s developers somewhere at Georgia Institute of Technology want to give away cancers attackers for freely, or at least have others adopt bits and pieces of their code, so they’ve released it under an open source licence. With the help of a large group of researchers, they want to enhance the programme and prevent more people.
Free and Open Source Machine Learning
An open challenge to researchers to steal their code. In an effort to create a powerful software application for the collective good, they are daring others to defeat them at their own game. The best potential advantage for patient care will be achieved via the free availability of not just labour but also fruits.
“We are unable to retain the information or code on our own or make money with it,” explained John Macdonald, head of Georgia Tech’s Integrated Cancer Research Center. If we leave it open, it will grow.
Participating researchers may access the latest study here; it was published in PLOS One per October 26, 2017. They may view the source code and download links for the programme there.
To get started, they’ll use a preexisting software that accurately predicts the success of nine medications based on the genetic information of 273 individuals, or about 85% of the time. This is shown and clarified in detail by the research conducted by McDonald and his colleague Fredrik Vanburg.
Free and open-source deep learning samples
Assistant Professor Weinberg from Georgia Tech’s Institute of Biological Sciences stated, “There are nine medications in the published paper, but we really run roughly 120 medications through the software.”
The software normalises data using tried and true machine learning algorithms. The latter makes it possible for machine learning to reconcile data from various sources.
Further, scientists have reduced the influence of bias when choosing which data to use in forecasting. To paraphrase McDonald: “It is more efficient to lower the burden of original information and permit the program solve it.” You shouldn’t go into the data with a preconceived notion of what you want to find since “it searches for associations, not reasons.”
The researchers’ bias came from their exclusive focus on gene expression data relevant to the cancer subtype they were trying to cure. According to Wanberg, “it shows out it is preferable to provide the computer data from a larger range of malignancies,” which will ultimately provide a more accurate prediction of drugs’ efficacy against particular tumours like breast cancer.
Tensorflow
Molecularly speaking, “certain mammary cancers, for illustration, maybe more comparable to some malignant tumors than other cancer cases,” McDonald said. We fed all of the data into the programme, and it produced highly accurate results.
Researchers also want to amass a sizable quantity of anonymized diagnosis and treatment successful and unsuccessful data to aid the software in fine-tuning its estimates for the betterment. However, this does not rule out the possibility that certain businesses may reap rewards.
Read Also: 300 Neurons Traced in Extensive Brain Map
Professor McDonald from Georgia Tech’s School of Biological Sciences stated, “If a corporation utilises the programme to appear with patients and generate gain, that’s OK, since there is no requirement to pay up the project.” If that happens, more people could start using the bus as an alternative. However, I’m keeping my fingers crossed that the majority of gamers will feel some compassion for the situation.
We want to spread the message that sharing is good for everyone via this effort. Algorithms for open-source machine learning in cancer medication therapy prediction
Machine Learning for Breast Cancer Prediction
The subject of accurate medicine is thriving in the current medical sciences, and open-source computer code is crucial to the advancement of standardised and controlled study of patient records. Predicting the best course of medication treatment based on the genetic profile of a patient’s tumour is a critical part of effective cancer therapy.
In this work, we provide a free and open-source software platform for predicting individual medication sensitivities from expression profiles of genes using a highly flexible support vector machine (SVM) technique and a typical recursive feature elimination (RFE) technique.
Utilizing gene expression and medication responder information from the National Cancer Institute’s board of 60 human carcinoma cell lines, drug-specific models were developed (NCI-60). The models perform very well in identifying therapeutic efficacy for a wide range of carcinoma cells, namely those used in the most recent iteration of the NCI-DREAM Challenge.